About
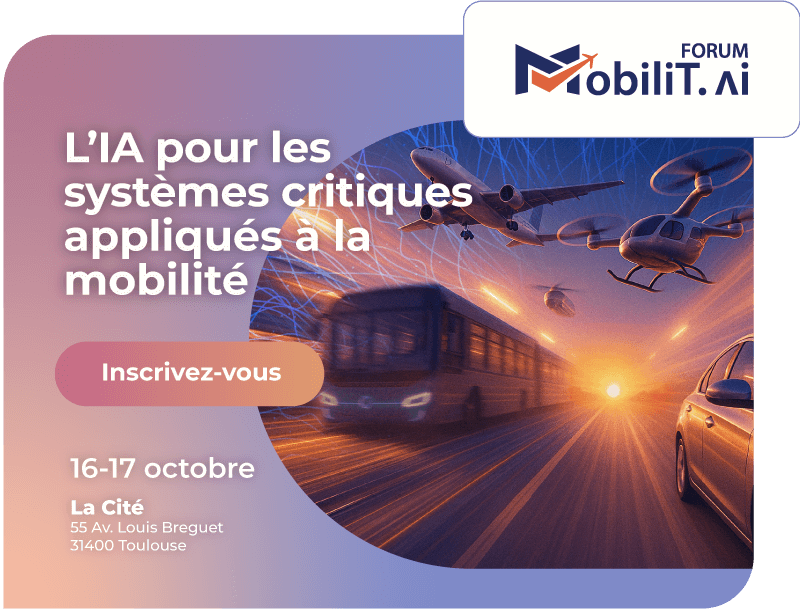
IVADO,IRT Saint Exupéry, IATA,IID, CRIAQ and ANITI are organizing the 6th edition of the MobiliT.AI forum on October 16 and 17, 2025.
MobiliT.AI: this international event brings together a community of experts in Artificial Intelligence for mission-critical systems in the field of transport and mobility (aeronautics, automotive, rail, space, drones, etc.). This community is made up of academic and industrial researchers and Tech companies, as well as experts in operational safety and critical embedded systems engineering.
More information on previous editions: